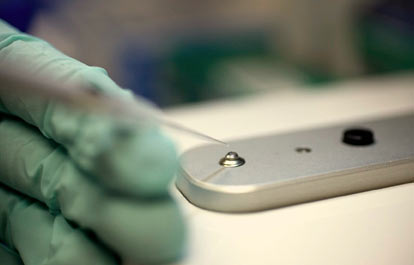
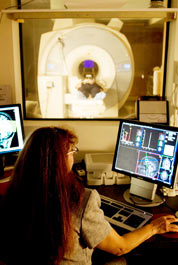
Scientific Lectures //
Incremental Robust Principal Component Analysis for Video Background Modeling: Theory,...
“Incremental Robust Principal Component Analysis for Video Background Modeling: Theory, Applications and Jitter Invariant Extension”
Paul Rodriguez, Ph.D., Professor, Department of Electrical Engineering, Pontificia Universidad Católica del Perú (PUCP)
Presented: November 11, 2016
ABSTRACT: While Robust Principal Component Analysis (RPCA), a.k.a. Principal Component Pursuit (PCP), is currently considered to be the state of the art method for video background modeling, it suffers from a number of limitations, including a high computational cost, a batch operating mode, and sensitivity to camera jitter. The original PCP problem considers the nuclear and l1 norms as penalties for the background (low-rank) and foreground or moving objects (sparse) with an equality constrain for the observed videos and low-rank and sparse components. In this talk we propose to change constrains to penalties, obtaining a variant where the restoration error (observed video minus low-rank and sparse component) and l1 norm are penalties while imposing the rank of the low-rank component as a constraint. Interestingly, this particular variant can be effectively solved in an incremental fashion, allowing real-time implementation for live-feed HD videos; moreover, considering T(.), an unknown rigid transformation, applied to the low-rank component, we can also cope with translational and rotational jitter, allowing almost real-time processing. Furthermore, in this talk we will also include a detail analysis of the proposed RPCA variant as well as incremental SVD, which is the key to solve the equivalent problem incrementally.
To view this presentation please click here.