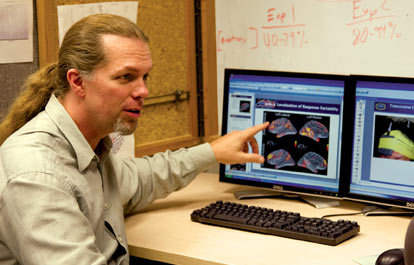
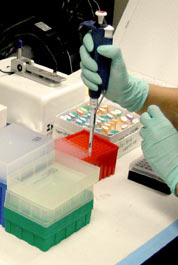
Scientific Lectures //
Density Functional Estimation
Barnabás Póczos, Ph.D., Assistant Professor, Machine Learning Department, Carnegie Mellon University
Presented: September 29, 2017
ABSTRACT: A crucial challenge at the interface of machine learning and nonparametric statistics is that many fundamental problems include the estimation of density functionals such as i) the randomness of random variables, ii) the dependence between random variables, and iii) the distance/divergence between distributions . While information theory provides standard tools to measure these quantities (Shannon, Rényi, Tsallis entropy, (conditional) mutual information, Kullback-Leibler, Bregman, Rényi, L2 divergence/distance, etc.), it is still unknown how to estimate these quantities from samples in the most efficient way under various conditions. In this talk we review possible estimators for these quantities and discuss their statistical properties.
To view this presentation please click here.