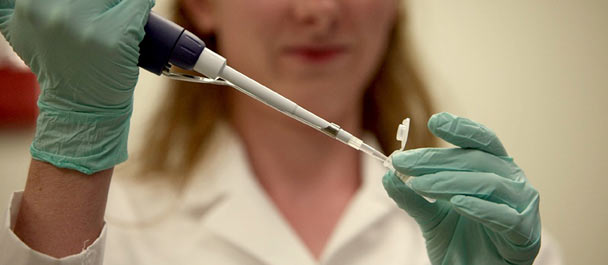
Medical Image Analysis //
Medical Image Analysis Lab
The Medical Image Analysis (MIA) lab is one of the largest labs at MRN. With more than thirty researchers from diverse backgrounds (electrical engineering, computer science, physics, math and statistics) and with the help of multiple multimillion dollar grants, MIA lab’s main research focus is to develop methods to better understand brain structure, function and human behavior. The MIA lab has developed a suite of powerful software tools to help other researchers investigate different types of data sets: sMRI, fMRI, DTI, EEG, MEG, genetic data, among others. Below are a few of the research areas in which the MIA lab is involved.
Methods to identify brain imaging biomarkers: Each brain imaging modality reports on a different aspect of the brain (e.g. gray matter integrity, blood flow changes, electrical activity) and each has strengths and weaknesses. Many mental illnesses, such as schizophrenia, bipolar disorder, and depression, currently lack definitive biological markers and rely primarily on symptom assessments for diagnosis. Methods developed by the MIA lab help to find multimodal biomarkers to characterize and classify complex brain disorders such as schizophrenia. Novel features with high consistency are found applying multivariate methods which focus on higher order statistics such as independent component analysis (ICA). A major focus of the lab is incorporating available knowledge (such as spatial or temporal constraints) into these algorithms in order to improve performance.
Spatiotemporal fusion of imaging and genetic data: Each existing modality for imaging the living brain can only report upon a limited domain. For example, functional imaging provides information about dynamic blood flow changes in response to a stimulus, whereas electroencephalography (EEG) provides information about the electrical activity of the brain with centimeter spatial and millisecond temporal resolution. Finally, gene array imaging can assess specific differences at the chromosomal level that are present in individuals, some of which have functional consequences. Multiple imaging modalities are typically collected on the same set of individuals and methods to effectively combine these different types of information are developed by the MIA lab.
In addition to the above main research areas, MIA is also interested in the following areas: analysis of complex-valued fMRI data, development of data driven methods for brain image analysis, imaging genetics, image based classification and machine learning tools, and others. For further information about the MIA lab, to download software tools developed by MIA lab and for individual research interests of MIA lab’s researchers please visit the lab website.
Research //
- Neuroimaging >
- Exploration and Exploitation of MRI Phase Data >
- 4D Magnetic Susceptibility Tomography >
- BOLD fMRI Simulation >
- Multimodal Data Fusion for Brain Connectivity Analysis >
- Capturing Complex Interactions in Neuroimaging Data >
- Multivariate Analyses on Genetic Effects on Brain Abnormality Associated with Schizophrenia >
- Exploring similarity and differences among schizophrenia and bipolar disorder by combining fMRI and >
- Fusing multi-task and multi-modal brain imaging data and indentify the potential biological markers >
- Imaging Genetics on Huntington’s Disease >
Neuroimaging
Incidental findings in neuroimaging research. Neuroimaging provides a tremendous amount of information to the researcher. It also uncovers findings in approximately a third of all research subjects that may not be related to the original research question, but may have medical relevance for the individual. Ethical principles need to be considered when deciding how much and by what method research information is offered directly back to subjects who volunteered for the research project. Our multidisciplinary collaborative group is studying the effect of providing all research subjects with an official reading of their MRI scan, probing relationships between key ethical principles such as beneficence, subject autonomy and justice. Our goal is to contribute to the national discussion regarding incidental findings in research.
Exploration and Exploitation of MRI Phase Data
The output of T2*MRI is a complex-valued MR image. Conventionally, the MR magnitude images are adopted for brain imaging (structural and functional) and the MR phase images remain unused. This project aims to understand the imaging mechanism of T2*MRI and to explore ways making use of the MRI phase data for brain imaging.
4D Magnetic Susceptibility Tomography
Our recent research shows that the MR magnitude image is not a replica of inter magnetic susceptibility distribution (the underlying vascular source of T2*MRI) and the magnetic susceptibility source can be reconstructed by a computed inverse MRI (CIMRI) technique. This project aims to reconstruct a 3D internal magnetic susceptibility source distribution (3D magnetic susceptibility tomography) from a T2*MRI phase image and to reconstruct a 4D magnetic susceptibility dataset (4D magnetic susceptibility tomography) from a BOLD fMRI dataset and advocate to upgrade the biomedical tissue imaging and neuroimaging based on the reconstructed magnetic susceptibility database.
BOLD fMRI Simulation
This project aims to look inside the BOLD fMRI mechanism (from the neurovascular coupling to multivoxel T2* imaging) by numerical simulations and numerical characterization. Typical topics include: 1) MR magnitude and phase behaviors with respect to image resolution, echo time, vasculature, field strength, diffusion effect, etc; 2) Morphological mismatch between the magnetic susceptibility source and MR image; 3) Nonlinearity of T2*MRI; 4) Isotropic diffusion in gray matter and anisotropic diffusion in white matter; 5) Computed inverse MRI (CIMRI); and 6) 3D and 4D magnetic susceptibility tomography.
Multimodal Data Fusion for Brain Connectivity Analysis
Despite enormous strides in our understanding of neural physiology, the transition from cellular and subcellular functional variability to variations in human behavior is poorly understood. Current evidence ties this transition to complex interactions within brain functional networks. These networks are not easy to estimate from the data due to their dynamic nature. However, accurately characterizing them is of the uttermost importance for diagnosis and prediction of mental disorders at their early stages (e.g. schizophrenia). This project is aiming to improve estimation of brain functional networks by taking advantage of the complementary nature of multiple brain imaging modalities. The main application is in the study of mental disorders linked to brain network dysfunction (schizophrenia, Alzheimer's, ADHD, bipolar disorder and others).
Capturing Complex Interactions in Neuroimaging Data
Unsupervised data analysis approaches have been widely used in recent years and have become instrumental in establishing new research directions impossible with more traditional supervised approaches, such as the study of the default mode network of the brain. Growing interest in unsupervised analysis of multi-modal data, multi-subject studies, whole brain activit and other datasets involving multiple interacting variables, have increased demand for multivariate unsupervised techniques. However there is still relatively little work on the examination of the full relationships among interacting variables in an unsupervised fashion. In this project, we work on a novel approach to address the problem of identifying these higher-order interactions. Unlike existing approaches, we directly identify sets of inter-dependent random variables without explicitly modeling interactions within these sets.
Multivariate Analyses on Genetic Effects on Brain Abnormality Associated with Schizophrenia
Multivariate analyses on genetic effects on brain abnormality associated with schizophrenia: Genetics play an important role in psychiatric disorders including schizophrenia. Not a single gene but many genes interact together, and contribute to the risk of development of schizophrenia. We have developed and continue to develop multivariate methods to assess the complex genetic effect on brain based on phenotypes associated to schizophrenia.
Exploring similarity and differences among schizophrenia and bipolar disorder by combining fMRI and
This project will develop an exploratory data fusion model which combines 2 multivariate methods and is able to identify correspondence among multiple data types. We aim to apply this model to schizophrenia and bipolar disorder via an fMRI-DTI fusion, which can identify both shared and disease-specific brain abnormalities from multiple perspectives (brain function and structure).
Fusing multi-task and multi-modal brain imaging data and indentify the potential biological markers
Each brain imaging modality reports on a different aspect of the brain with different strengths and weaknesses and there are now literally thousands of putative imaging biomarkers. This project will develop multivariate methods which use higher order statistics to combine diverse information in a scalable manner, identify correspondence among data types and also provide a sophisticated data sharing and management system.
Imaging Genetics on Huntington’s Disease
We will use our imaging genetics analysis methods in the PREDICT HD dataset to identify structural networks and genetic profiles which relate to the predicted time to disease onset. We use cross-sectional data to identify these patterns, and use the longitudinal data to identify which of these imaging genetic patterns predict the rate of loss of function. A recently released Huntington's Disease Signaling Pathway identifies genes which interact with the Huntington gene HTT, and forms a high-priority geneset to examine. We exepect that cumulative effects of many genotypic factors from this pathway in conjunction with structural "fingerprints", identified using independent components analysis ICA, will account for additional variance in disease progression (pre-diagnosis) in the lower-repeat subset of the prodromal HD sample.