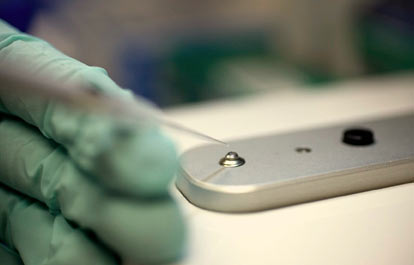
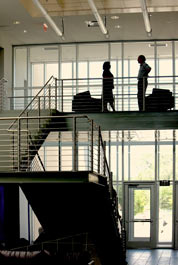
Scientific Lectures //
The Statistical Bootstrap, an Introduction with Examples
Erik Erhardt, Ph.D. - Professor, Department of Mathematics and Statistics, University of New Mexico
Presented: December 16, 2014
ABSTRACT: Uncertainty is central to statistics. Uncertainty is formalized with prior information, probability models, likelihood, standard errors, and confidence intervals (CIs). In simple situations, the uncertainty of an estimate may be based on an assumed probability model for the available data. In more complicated situations this approach may be tedious and difficult, and results may be misleading if inappropriate assumptions or simplifications are made.
Bradley Efron's bootstrap (BS) obtains reliable standard errors, confidence intervals, and other measures of uncertainty for a wide range of problems without making specific distributional assumptions. The key idea and strategy is to: (1) regard the sample of observations (data) as an accurate representation of the population; (2) resample from the data (either directly or via a fitted model) to create replicate bootstrap resample datasets from which the variability of the quantities of interest may be assessed; and (3) construct BS confidence intervals (CIs) for parameter inference or calculate BS p-values under the null hypothesis for making hypothesis test decisions. Furthermore, in the spirit of robustness of validity, the BS can be used to check results given a particular parametric model.
The bootstrap uses the data to generate more "data", analogous to a trick used by fictional Baron von Munchausen, who when he found himself at the bottom of a lake got out by pulling himself up by the bootstraps.