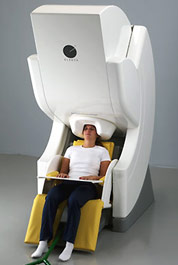
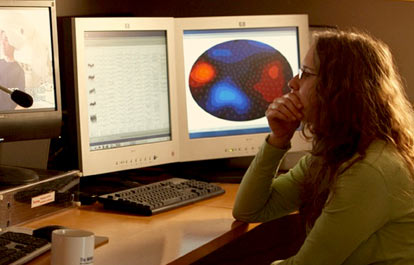
Scientific Lectures //
Sparse Representation Based Methods for Integrative Imaging and Genetic Data Analysis
Dongdong Lin- Department of Biomedical Engineering at Tulane University
Presented: October 10, 2014
ABSTRACT: Imaging genetics, aims at integrating imaging and genomic data to enhance our understanding of complex diseases in a systematic manner. However, high dimensionality and heterogeneity of these datasets present a significant challenge to statistical analysis. In this talk, I will present our recent works on developing sparse representation based approaches to address this challenge. I will present several sparse models for multi-modal analysis such as sparse canonical correlation analysis for exploring the correspondence between imaging endophenotype and genetic factors. I will show how to develop sparse group-ridge low-rank regression and collaborative sparse group lasso regression models and apply them to the association analysis between multi-locus genetic factors and multiple correlated imaging phenotypes. At the end, I will present a generalized sparse model to integrate genetic, imaging and disease status for detection of risk genes and classification of complex diseases such as schizophrenia.
BIOGRAPHY: Mr. Dongdong Lin received the BS degree in Biomedical Engineering from Chongqing University in 2007, and the MS degree in BME from Tianjin University in 2009. He is currently a Ph.D. student in BME at Tulane University. He is also a member of Tulane Center of Bioinformatics and Genomics. His research interests have been computational system biology, bioinformatics and machine learning for integrative analysis of multiple omic datasets and medical imaging data.