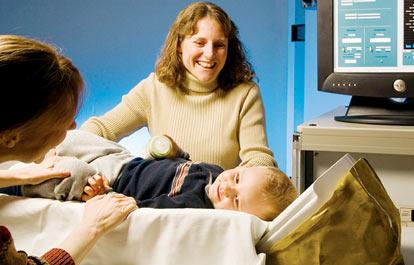
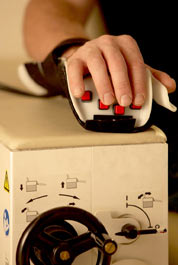
Scientific Lectures //
Alexa, Analyze My Data: Things to Consider When Automating and Interpeting MRI Data
Thomas DeRamus, PhD, Postdoctoral Fellow, The Mind Research Network
Presented: February 26, 2019
ABSTRACT: There are a multitude of data quality, pre-processing, and analysis pipelines for neuroimaging data, many of which perform similar operations using different methods. While consistently utilizing pipelines has the advantage of reproducibility across datasets, pipeline customization is increasingly being utilized within the neuroimaging community as some software(s) may outperform others at different stages of data processing or analysis. Multi-software pipelines such as FMRIPREP, packages such as Nipype (python) and Neuroconductor (R), and workflow generators such as Porcupine and Giraffe have made these “mixed” pipelines easy to create.
However, accurate processing and analyses from neuroimaging pipelines still require nuanced knowledge of the underlying data, including but not limited to: data quality, population of interest, acquisition parameters, nuisance regression strategy, and distribution of the data. Furthermore, there has been considerable discussion regarding a lack of statistical power within neuroimaging data, but very little discussion regarding spurious results which may be produced by very large sample sizes. Identifying such results require a modest understanding of sources of artifact in MRI, appropriate methods to calculate effect sizes for models utilized to analyze imaging data, and conclusions which reflect the utility of results as opposed to their statistical significance.
This abstract provides a literature review of modeling concerns that have been raised regarding MRI data pipelines over the last 5 years and the tools and concepts to address each. The goals of the presentation are to introduce these concepts to individuals new to MRI analysis, foster discussion with experts in the field regarding agreement or disagreement with these trends, and/or how to address these issues in the increasingly protean field of neuroimaging research.