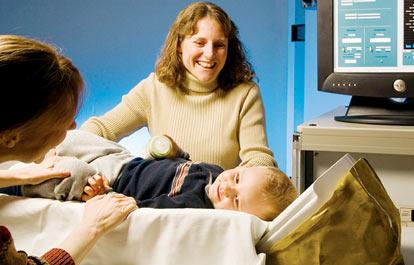
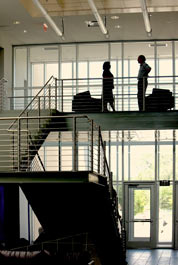
Scientific Lectures //
Enabling Collaborative Research with Privacy-Preserving Machine Learning
Anand Sarwate, PhD. - Assistant Professor, Department of Electrical and Computer Engineering, Rutgers, The State University of New Jersey
Presented: April 22, 2014
ABSTRACT: Advances in imaging and biomedical testing technologies have led to an explosion of healthcare data. At the same time, providers are implementing electronic medical record systems to manage and standardize this data. This confluence can potentially transform the way we understand healthcare data by allowing researchers to learn from existing clinical data, share data from experiments, and perform large-scale comparative effectiveness analyses. Privacy concerns are a major obstacle to overcome before enabling these goals. There has been a flurry of recent work on new models for privacy risk and protection. Sharing raw data is often too risky; a different approach is to only share access to data derivatives. Differential privacy is a model that quantifies the risk of patient re-identification from sharing functions of the data. Differentially private algorithms guarantee limited privacy risk while performing useful computations on the data. In this talk I'll describe this privacy framework and how to use it for common research analyses such as cohort identification and classification.
To view presentation please click here.