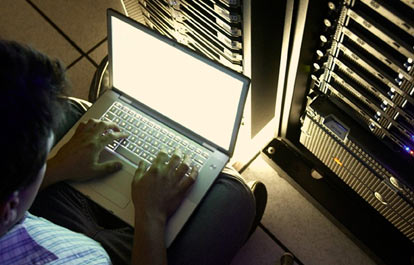
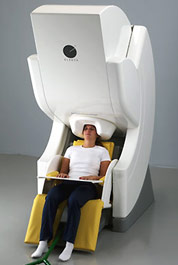
Scientific Lectures //
Predicting Clinical Variables from Neuroimaging Data Using Structured Sparse Regression
Joanne C. Beer, MS, University of Pittsburgh, Department of Biostatistics
Presented: June 11, 2018
ABSTRACT: Predictive models in which neuroimage features serve as predictors and a clinical variable is modeled as the outcome are good candidates for clinical application because (1) they can exploit dependencies between predictor variables and thus potentially explain more variability in the outcome than a mass univariate approach, and (2) they allow inference at the individual level, such that a prediction can be obtained for a new individual whose data was not used to train the model. In this talk, I discuss methods for neuroimaging prediction models that not only aim for predictive accuracy, but also seek interpretability and potential insight into the underlying pathophysiology of psychiatric disorders. In the first part of the talk, I introduce the fused sparse group lasso penalty, which encourages structured, sparse, interpretable solutions by incorporating prior information about spatial and group structure among voxels. Simulation studies show conditions under which fusion and group penalties together outperform either of them alone. In the second part of the talk, I demonstrate application of fused sparse group lasso and other penalized regression methods (e.g., lasso, ridge, elastic net) to predict continuous measures from resting state MRI data using the Autism Brain Imaging Data Exchange dataset, and to predict total brain amyloid load and age from multimodal imaging data drawn from a normal aging study. In general, I show how the incorporation of prior information via the fused sparse group lasso penalty can enhance the interpretability of neuroimaging predictive models while also yielding good predictive performance.